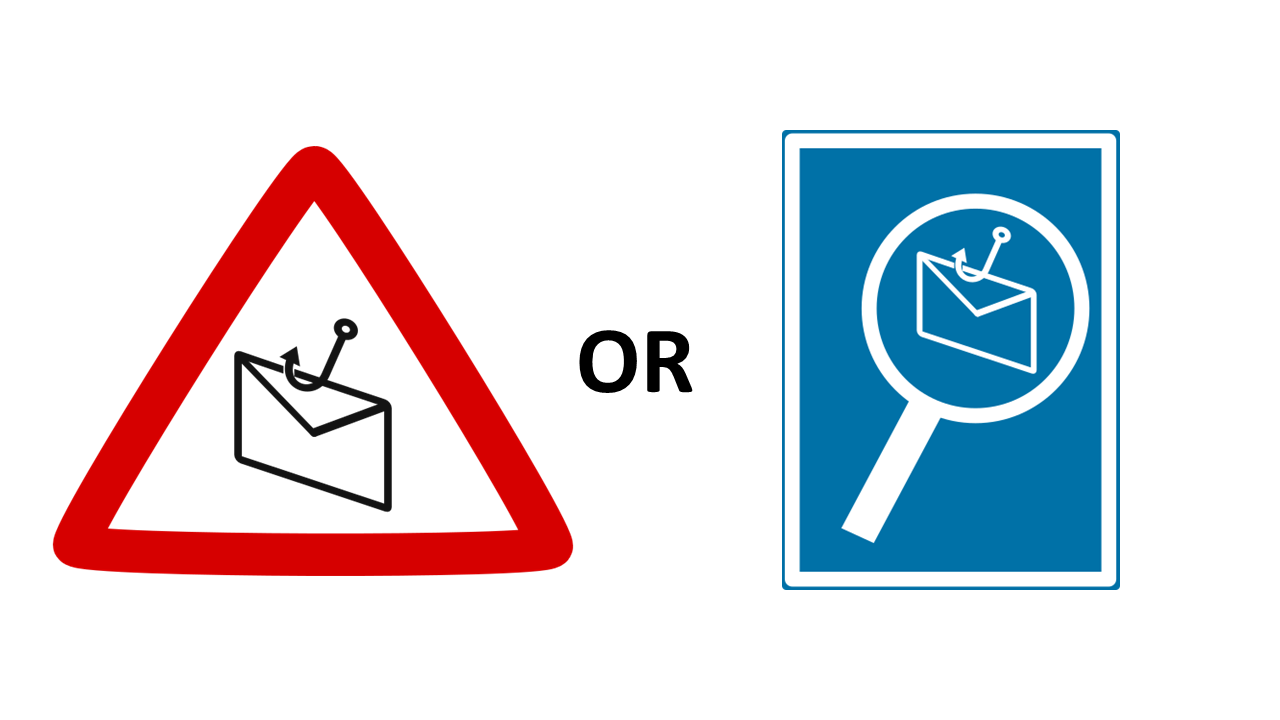
Some questions seem to have no definitive answers. The egg and the chicken is one of them, and within the small world of Phishing, KPI or KRI is another one.
The question seems trivial. Do we consider the risk or the performance? Do we want to measure how many of our people will likely fail a phishing test or how many will detect it? It is the kind of question most people would likely dismiss. Just pick one, could we say. However, there is more to it than meets the eye.
We use Key Risk Indicators and Key Performance Indicators to help steer our company. They must provide relevant information allowing us to decide if we are on the right track, at the right speed and in the right direction or with the right level of protection. The burn rate and the net profit margin are standard financial KPIs. They allow us to know where we are going financially. What kind of indicator would best achieve the same objective when it comes to Phishing?
Phishing is a risk for most, if not all, companies. If we consider the risk approach, using a KRI makes sense. We often use the click ratio to measure the risk of a company being vulnerable to a phishing attack. Risk officers usually calculate it using the number of people clicking on a phishing link divided by the number of people who received the email. It makes sense, no? No! Not entirely at least. First, it does not measure the actual risk. Second, it is not an accurate measure of the risk.
Let us take a closer look at the question.
First, clicking on a link in an email will most probably cause no harm. The danger comes afterwards when users disclose credentials on a phishing website or open a file they just downloaded from it. The other possible threat with a phishing email is to open a malicious attachment. It will allow the propagation of ransomware or the installation of malware.
If we want to measure phishing related risks, these three behaviours are our more relevant candidates. One could say that clicking on an attachment or a link is still clicking. True, but not true. Our researches, confirming others, shows that we can have a reduction in the likelihood of clicking a malicious link and, still, having many people opening attachments. If we do not train our users specifically to be vigilant with files, they will not be as cautious as with links. Consequently, we should have multiple risk indicators, one for credential disclosure, one for downloading and opening files, and one for opening attachments. A KRI can be build up using the average or the worse result of these three indicators.
Still, we would not have an accurate measure of these risks. As discussed in a previous post, the variance between scenarios is way too high to be able to extrapolate a risk exposure using one situation only. The only reliable result we will have from a test using one scenario is the likelihood of our users to fall for this particular scenario at that moment. Is it enough to make an informed decision? Unlikely.
Worse, we cannot predict with certainty which scenario will have more impact. In other words, the margin of error of such measurement is probably around 40%. We can easily agree that we cannot rely on such an uncertain result to take any decision. Somehow, we should probably accept that measuring our risk exposure is difficult and move to another indicator.
Instead of the risk of failing, we could use our performance in detecting, and reporting, phishing email as an indicator. We could think of the performance in detection as the opposite of the failure. That would be a mistake. Let us have a look at the split of possible behaviours when people receive an email. We can see on the pie that there is a large part of it that is neither green (Detection and reporting) nor red (failure).
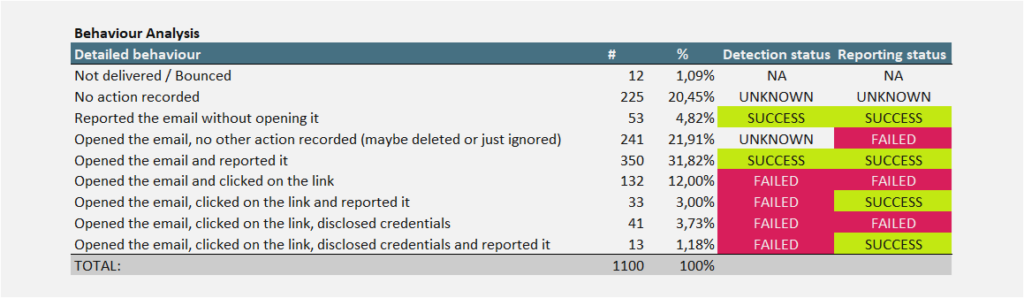
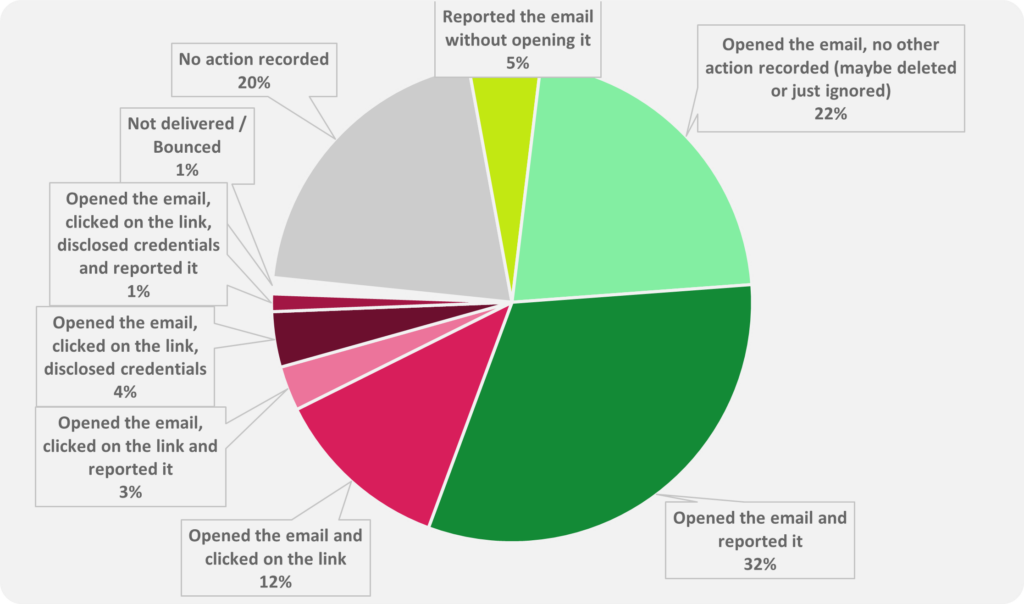
The number of people reporting phishing emails is not complementary to the number of people failing the phishing exercise. First, we can fail the test and still report. We should even make that mandatory. It shows that, despite having failed the exercise, we have understood it is essential to pay attention and to report. It emphasizes that accidents may happen, but we still have to perform the expected behaviour.
Regarding the Unknowns, keep in mind that the subject could have opened the email and just deleted it or ignore it. He may believe that it is a genuine email, and might process it later.
The scenario will also have a significant impact on the result. When the scenario is more relevant for the targeted population, there is more chance that the people will fail or detect it as more people will open it.
We can summarize these results in two simple graphs focusing on the risk (clicking) and the performance (reporting):
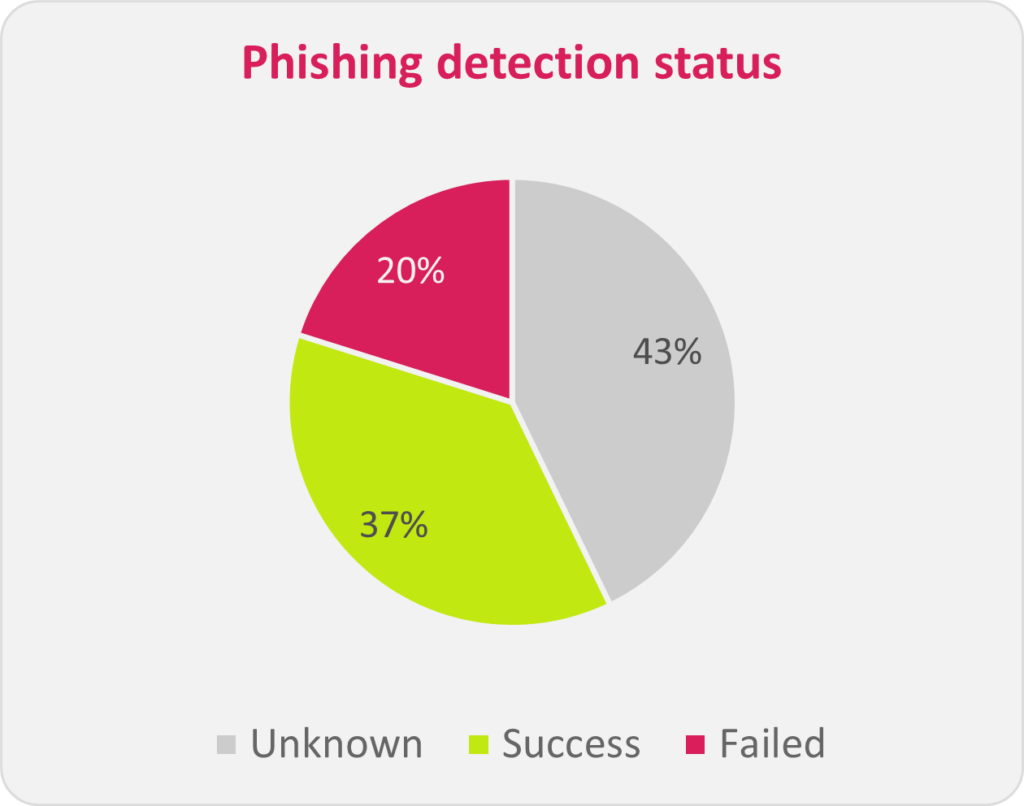
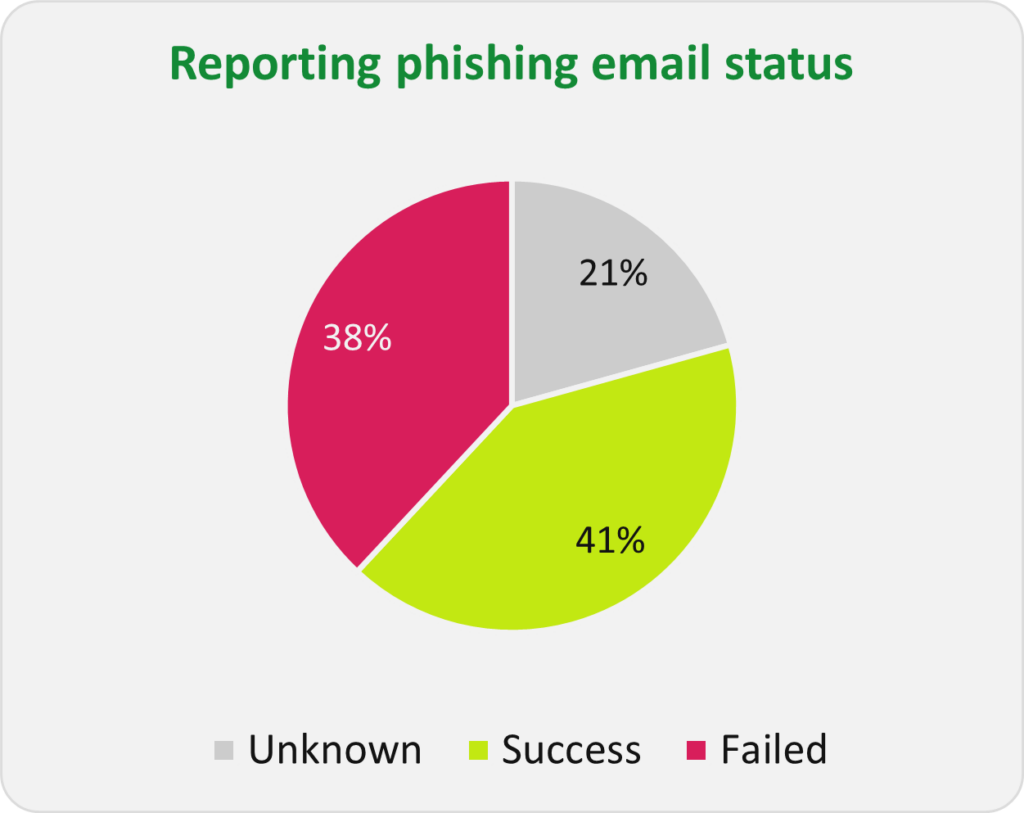
We should also measure the performance using the ratio between the number of people reporting the phishing email divided by the number of people opening the email. That gives us an accurate view of the percentage of people in our organization performing the expected behaviour, whether they were able to detect the phishing exercise or not.
The email opening ratio will also provide insights on the scenario relevance. The first challenge for a phishing email is to stand out from the possible hundreds of emails received by the target and be read. The fate of many phishing email is just to be ignored. And, unfortunately, in such case, it neither trains the user neither provides us any good indication of their phishing susceptibility. So, the failure is more on the phisher / trainer side. Let’s also keep in mind that we might have some issues with false positive and false negative as the usual technique used to measure email opening (the white pixel trick) is not always reliable.
There is no perfect way of measuring the risk related to Phishing. However, the four scenarios protocol discussed in our previous post gives us a reliable measure of the effectiveness of training. It is the right candidate for a Key Indicator. Measuring the ratio of phishing reports also provides relevant information regarding Phishing education and cybersecurity culture. We should use both indicators while keeping in mind what they measure. If we read an instrument in a plane and misinterpret the value, it can lead to an accident. It could also happen with our Key Indicators.